B2B marketing teams invest significantly across campaigns and content to drive qualified website traffic. However, benchmarks find that only about 2% of this traffic actually converts, with the majority of visitors simply bouncing off or browsing anonymously on the website.
Retargeting the remaining 98% of visitors via LinkedIn has proved to be an effective strategy to recapture interest from anonymous website traffic. While we won’t cover the specific benefits of LinkedIn retargeting here, this case study summarizes how Sage successfully leveraged LI retargeting to:
- Generate 700,000 impressions in 6 weeks
- Improve lead generation by 4x
- And reduce cost-per-lead (CPL) by 80%
Simply put, LinkedIn retargeting works.
LinkedIn retargeting relies on the LinkedIn Insight tag (aka LinkedIn Pixel) to match website visitors with LinkedIn audiences.
The LinkedIn insight tag is a simple piece of code placed on a website to help optimize campaigns. While the LinkedIn Pixel serves many functions, including conversion tracking and demographic insights, it’s challenged by shortcomings around website retargeting.
While LinkedIn Pixel works to some extent, we have found an alternate approach that can take your retargeting campaigns to the next level, also known as account-based retargeting. Account-based retargeting works by identifying, qualifying, and targeting anonymous accounts, as opposed to individual users visiting a website. Using a combination of identifiers, account-based retargeting has been shown to deliver:
- Larger, account-level audiences
- Improved match rate accuracy
- Better segmentation and targeting
And the results? Well, they speak for themselves:
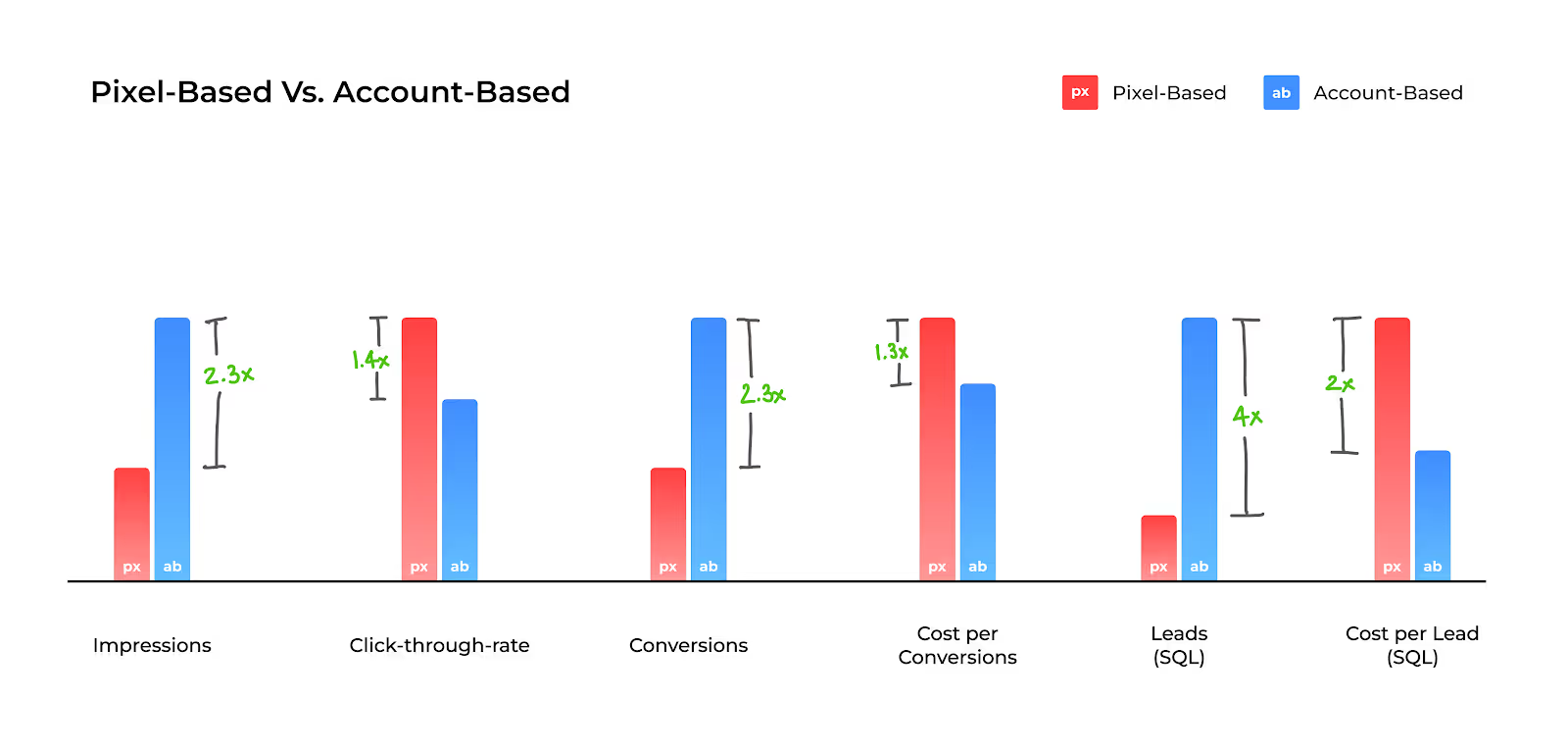
While the LinkedIn Pixel is a must-have solution given its wider functionality, we explore the limitations of Pixel-based retargeting and why Account-based retargeting is an effective alternative ⬇️
Limitation #1 - Match rates
The LinkedIn Pixel works by placing a cookie in visitors’ browsers, so when a LinkedIn user lands on your website, they may be identified and retargeted on LinkedIn. Note that this cookie-based identification takes place at a device and browser level for individual users. This means that, for the Pixel to match a website visitor to a LinkedIn user, the visitor must meet all 4 of the following criteria:
- Be an active member of LinkedIn
- Explicitly accept cookies on the website
- Use the same device (phone/laptop/tablet) to visit the website and LinkedIn
- Use the same browser (chrome/firefox/safari) to visit the website and LinkedIn
While a few visitors will probably fit this criteria, audience match rates via the Pixel are limited by the fact that the majority of traffic either doesn’t use LinkedIn, rejects cookies, or, most commonly, uses different devices/browsers for product research and LinkedIn browsing.
In fact, only about 42% of B2B product research involves mobile touch points — with the majority of B2B buyers choosing to conduct their research on desktops. On the other hand, a whopping 80% of LinkedIn engagement is via mobile. This is not surprising, given that LinkedIn is primarily a social networking app.
And so, despite the fact that LinkedIn Pixel works as designed, its match rates tend to be relatively poor, given the practical realities of B2B user behavior.
The limitation: Low match rates as a result of limited, cookie-based matching mechanisms by the LinkedIn Pixel.
How Account-based Retargeting helps
The LinkedIn Pixel relies exclusively on cookie-based tracking to create its matched audiences. Factors, on the other hand, leverages a combination of three identifiers — IP address, advertising ID, and cookies, to triangulate a data connection and match anonymous traffic to a company.
Factors connects with over 4.2B+ IP addresses and 65M+ company profiles (in addition to cookies and ad IDs) to accurately identify which accounts are visiting your website. Note that this is regardless of whether the visitor in question is a member of LinkedIn, uses different browsers, etc.
In fact, Factors can also identify remote companies by initially cookie-ing people using their corporate IP address to then re-identify them when they work remotely. To further explain how Factors achieves industry-leading match rates, here’s Viral from 6sense, one of our data partners:
“As a person moves around, their IP address changes. The platform adjusts for these changes by pulling in several additional markers to help match signals to an account. Now, with more variations in IP address data as remote working spreads across industries, our Graph deploys available secondary marker information, like cookies and mobile advertising IDs, to triangulate data connections. The Graph uses additional markers to sift through the noise so that confidence in the match rate remains consistent. Given the amount of signals we track, we don’t map every signal all the time, but we have observed accuracy over 85%.”
- CTO Viral Bajaria, 6sense, Data Partners
💡Build Better LinkedIn Retargeting Audiences with Factors
Limitation #2 - User-level targeting
B2B buying decisions are rarely made by a single person. The typical buying committee comprises almost a dozen people from multiple departments and time zones. Selling a SaaS product today might involve gaining buy-in from multiple C-suite executives, individual stakeholders from operations to sales to marketing, and a chief revenue officer – along with legal and implementation teams.
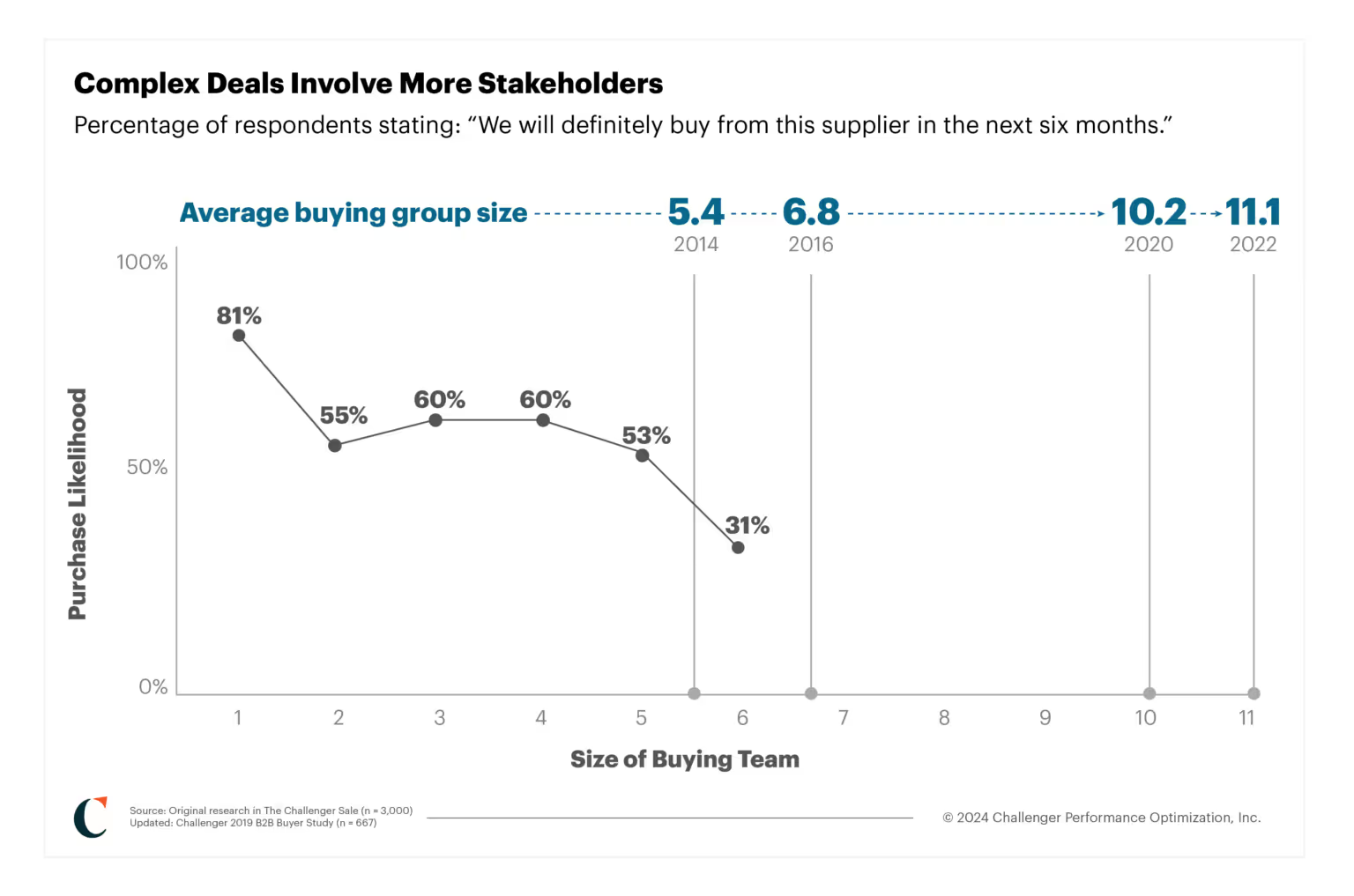
Source: Challenger
Given that the Pixel focuses on individual, single users visiting your website, it fails to capture the wider buying group from each account those users are from. This shrinks your total matched audience size considerably, but more importantly, it inhibits your marketing efforts from reaching key stakeholders and decision-makers who may not have been the ones visiting your site.
For example, if a junior marketer visits Factors.ai and is retargeted by the LinkedIn Pixel, the junior marketer alone will receive ads — with other stakeholders from the target account being ignored. As a result, this approach relies on the junior marketer being independently influential enough to convince the rest of the team to move forward with the deal. Definitely a tough sell.
The limitation: User-level targeting, as opposed to account-level targeting, results in fewer stakeholders targeted per account and smaller audience sizes.
How Account-based Retargeting Helps
While LinkedIn is best for targeting buying groups, it’s important to remember that there is no initial intent to buy on a social media platform. You need to layer in intent signals from multiple sources, such as your website and review sites like G2, to understand how you can best retarget relevant accounts.
Factors identifies intent signals and re-targets anonymous website traffic at an account level. This means that multiple decision-makers and stakeholders from the same company will be targeted on LinkedIn, regardless of which user actually visits the website. This bodes well for multiple reasons:
- Increases audience size without compromising on the quality of accounts
- Creates brand awareness at a company level rather than at an individual level
- Improves odds of targeting the right decision-makers within each account
Even assuming that account-based targeting finds the same 100 accounts as Pixel-based targeting, the former would generate an audience size of 300-500 users (3-5 people from each account), while Pixel-based targeting would only target 100 users (1 from each account). More importantly, a larger audience will improve the odds of targeting decision-makers, ultimately resulting in more leads and conversions. Accordingly, account-based retargeting solves for the practical limitation of LinkedIn campaigns struggling to scale due to poor audience size.
Limitation #3 - Audience segmentation
The previous two points discussed the LinkedIn Pixel’s limitations in terms of audience quantity. This third limitation highlights why the Pixel tends to fall short in terms of audience quality. In reality, a significant portion of your website traffic wouldn’t make a good fit for your business. Even within the subset of ICP accounts visiting your website, only a fraction would be “sales-ready” at any given moment (with the remaining accounts having to be nurtured until they’re prepared to buy).

As important as having a large audience is, the quality of this audience plays a key role in determining conversions and RoAS as well. In an ideal scenario, marketing teams should only retarget this subset of sales-ready “3000-pound marlin” accounts.
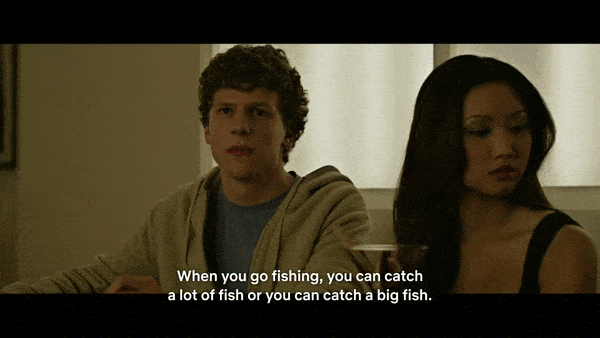
The LinkedIn Pixel limits audience segmentation based on intent and engagement. With the Pixel, website traffic can only be segmented based on page views. While this is definitely a good starting point, it lacks granularity.
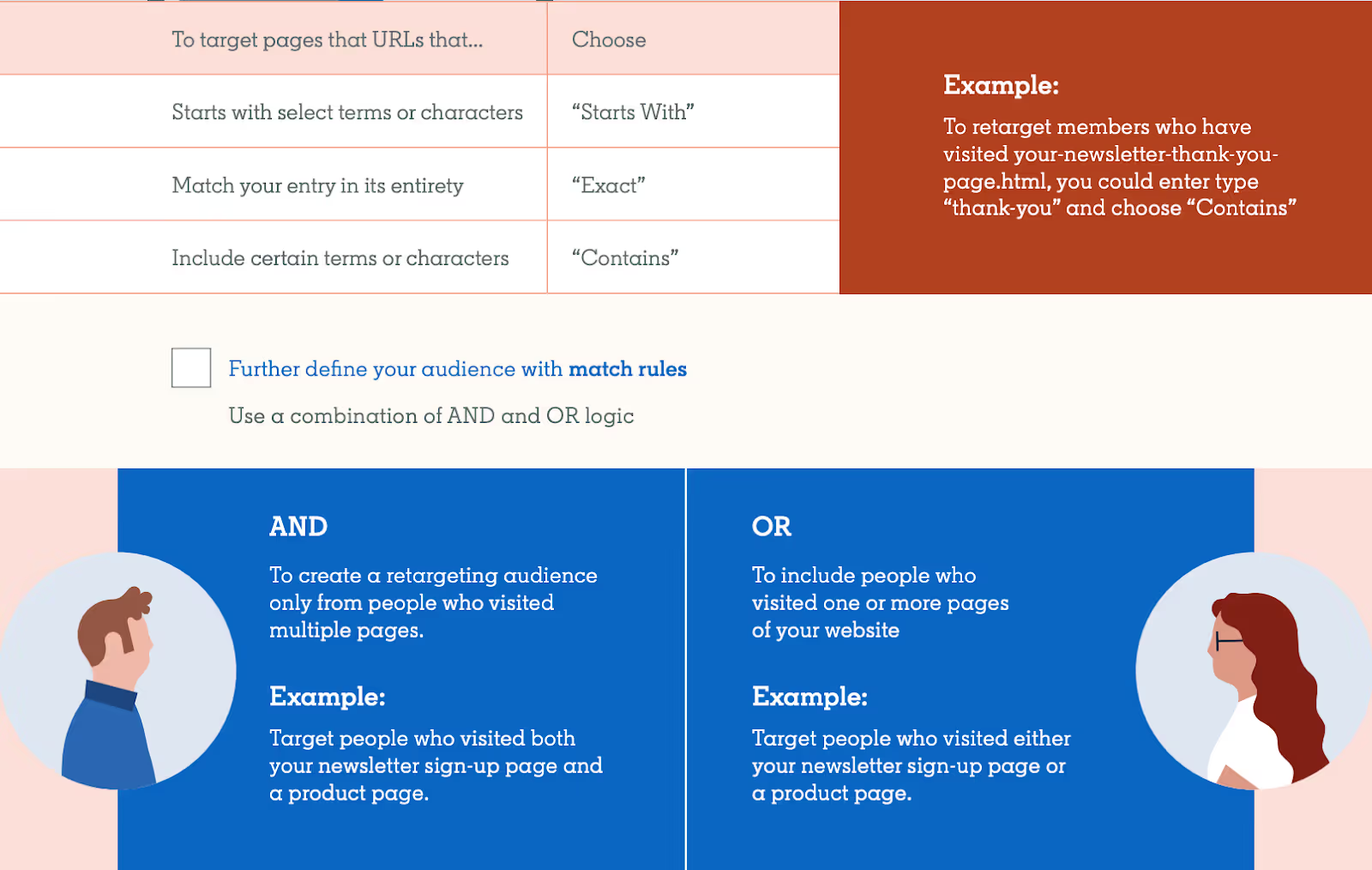
With Pixel, filtering out accounts that don’t match your target geographies, industries, sizes, or engagement levels can be challenging. This also translates to limited personalization options, as you can only segment campaigns by page views rather than by account and engagement properties.
The limitation: Limited segmenting & filtering options resulting in subpar audience quality and limited scope for personalization.
How Account-based Retargeting helps
Account-based retargeting with Factors supports granular segmentation based on a wide range of firmographics and engagement criteria. For example, with Factors, you can identify and retarget a list of accounts that meet the following rules:
“US-based Software companies with 100-500 employees visiting our pricing page & G2 profile for at least 10 seconds with a scroll-depth of 20% or more”
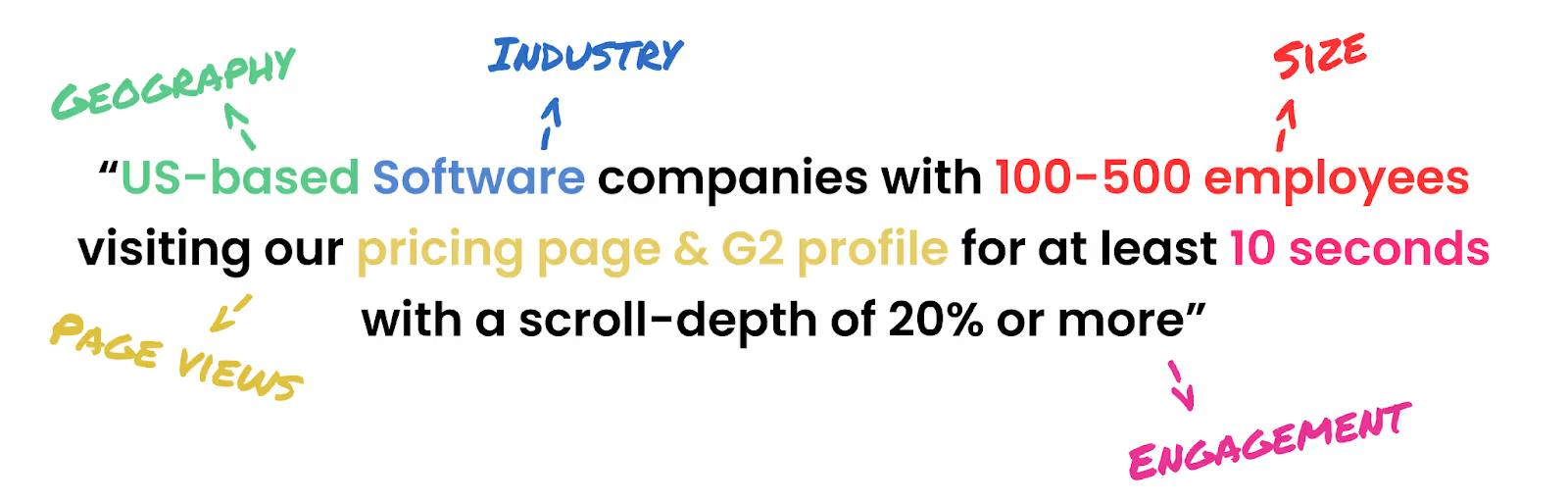
Here are a few ways in which Factors helps segment traffic data (in addition to regular old page views):
- Country
- City
- Industry
- Size
- Revenue range
- Time spent on page
- Scroll-depth
- Button clicks
- And a combination of all of the above
This level of filtering results in a list of precisely targeted ICP accounts that would make a great fit for your business. Additionally, by integrating your CRM, you may also include/exclude specific accounts, such as existing customers and competitors.
And guess what? We found the solution to fix your list-building problems once and for all!
Factors has launched Audience Builder, which allows marketers to automatically segment based on their preferred criteria, push these segmented audience lists to LinkedIn, and activate personalized, targeted advertising.
For example, you may choose to show accounts that visit high-intent pages such as factors.ai/pricing an ad creative offering a free trial. On the other hand, you can show accounts reading your competitor comparison blogs a “comparative” ad creative. The possibilities are endless.
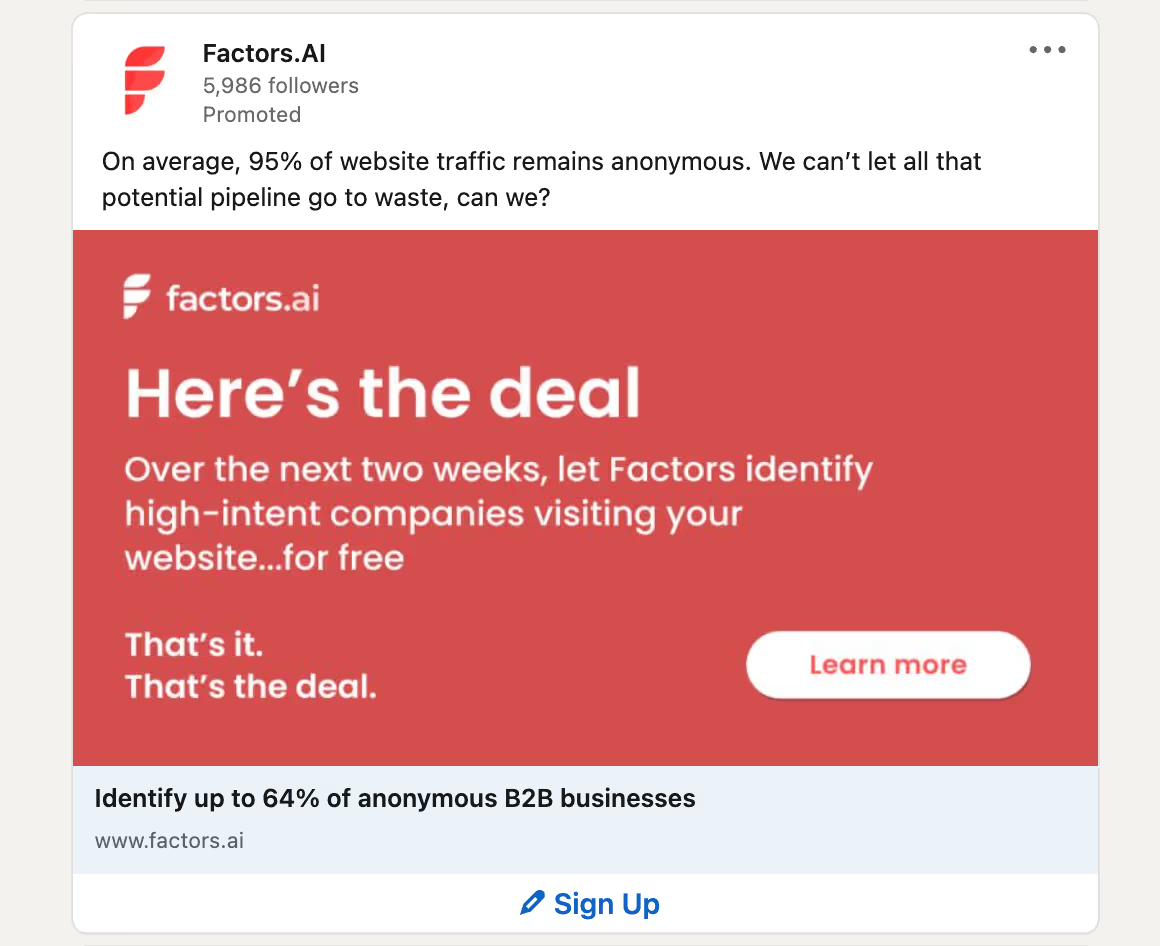
Real-life comparison: Pixel vs Account-based retargeting
We’ve talked the talk - now we’ve got the numbers to back it up. Here’s how two campaigns, one that’s Pixel-based and another that’s Account-based, compare to each other. Note that all else (duration, budgets, creatives, copies) has remained the same through the course of this experiment.
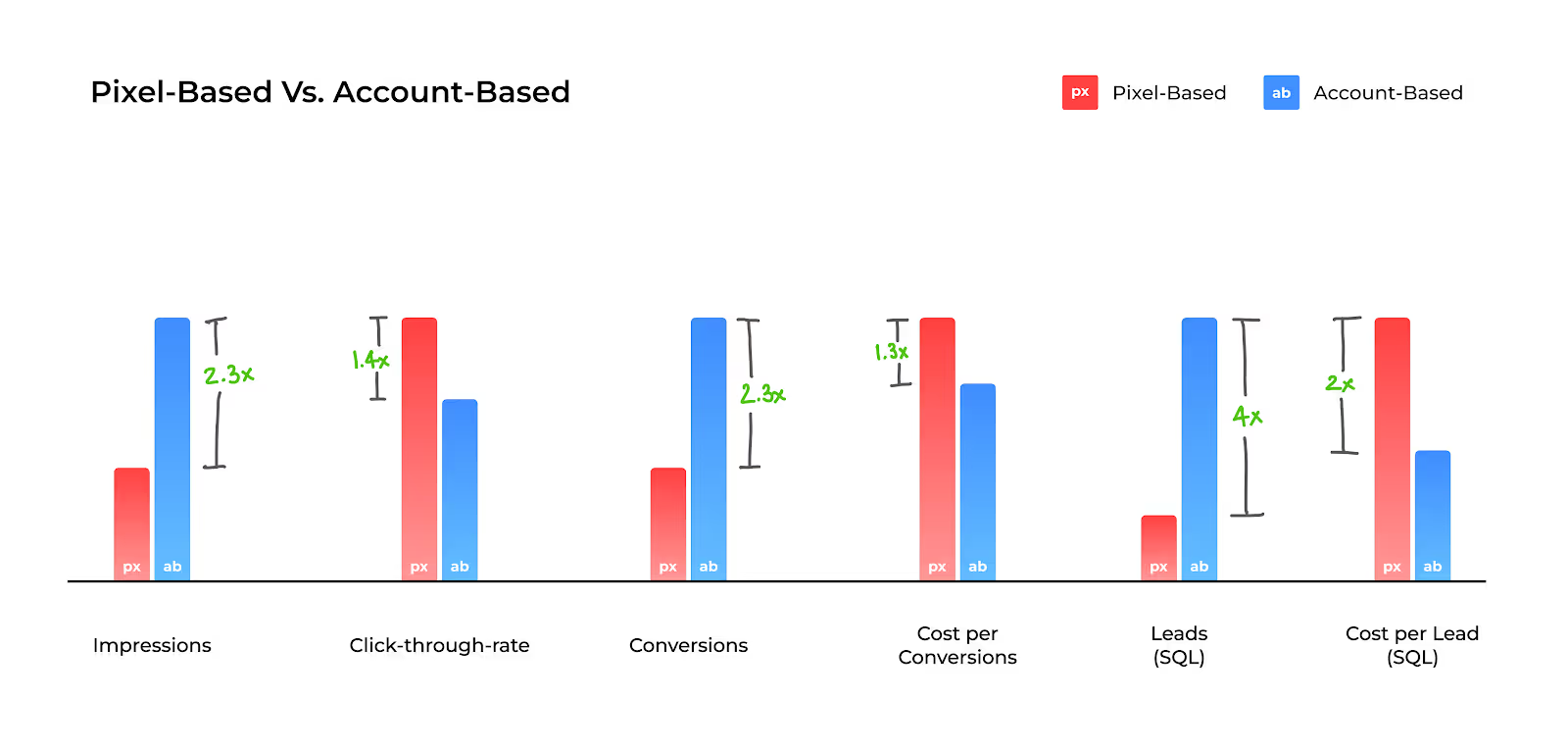
Over the same period, we find that CTR is higher under Pixel-based retargeting, likely because this approach targets exact users visiting the website. That being said, Account-based remarketing significantly outperforms Pixel-based retargeting in every other key metric, including leads generated.
And there you have it.
Solve your ad targeting woes with AdPilot
If you want to maximise ROI for your LinkedIn ads, look no further than our latest offering: LinkedIn AdPilot! We offer a wide range of features that allow you to segment audiences based on intent data, implement exposure control for your campaigns and determine the true ROI for your ads.
Speak to our team today to understand how you can use AdPilot to improve your LinkedIn retargeting efforts.