Sales and marketing teams struggle to identify and prioritize leads that are highly likely to convert. Manually scoring leads is time-consuming and often results in guessing and methodology inconsistencies.
What if there was a way to automatically score leads based on their conversion likelihood using data-driven insights and machine learning algorithms? This would allow teams to focus on the most promising prospects, personalize outreach, and improve conversion rates significantly.
The answer—predictive lead scoring.
Predictive lead scoring optimizes lead management by identifying leads most likely to convert using AI and historical data. This article will explore predictive lead scoring, its benefits, and how it transforms lead management strategies.
What is Predictive Lead Scoring?
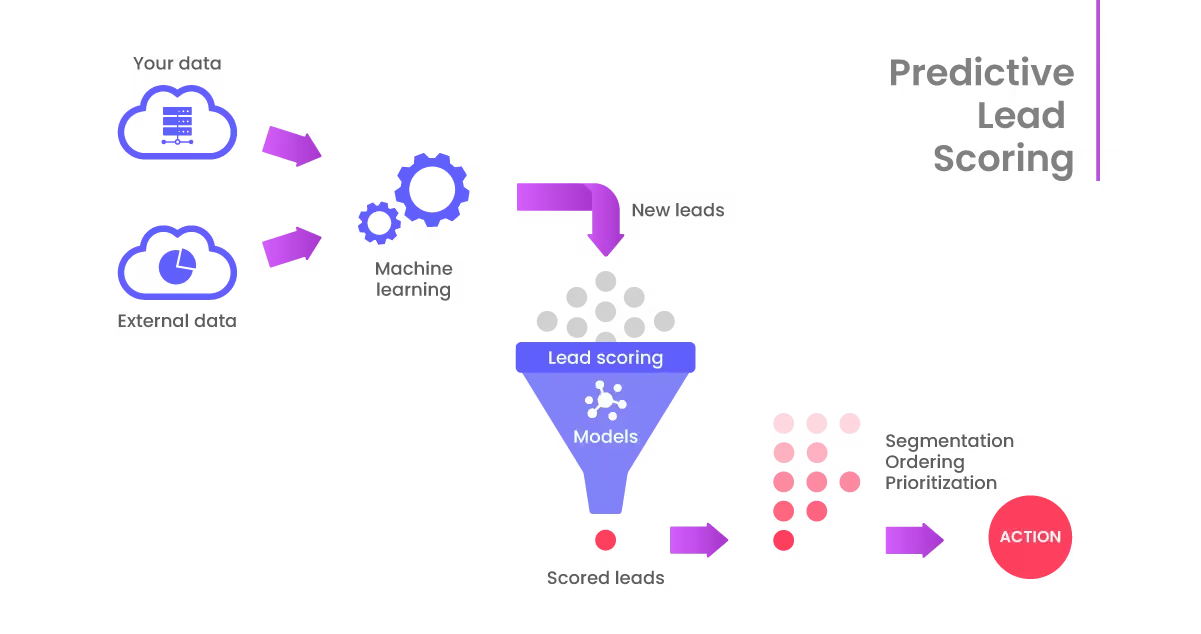
At its core, predictive lead scoring ranks leads based on their likelihood of converting into customers.
It’s a modern approach, evolved from traditional, manual lead-scoring methods.
Previously, sales and marketing teams manually assigned scores to leads based on factors like demographic information and behavior.
This approach was time-consuming and often relied on gut instincts rather than data.
But, as platforms became more advanced, so did their methodologies for lead scoring. In recent years, many platforms have also started using AI to power their lead scoring instead of rule-based scores.
Most lead scoring models use the following pieces of data to make sense of what stage of the buyer’s journey the lead could be at:
- CRM data
- Website behavior
- Social media interactions
- Email Engagement
- Demographic information
With this data, AI algorithms can identify patterns and correlations that indicate a lead’s likelihood of converting. You can use a lead scoring tool to identify and prioritize customers interested in your product.
The idea behind predictive lead scoring is to eliminate the unnecessary blockers that slows and help them make real-time decisions based on the right data. Let’s further explore the benefits of predictive lead scoring
5 Benefits of Predictive Lead Scoring
Predictive lead scoring can be quite powerful and lead to several impactful benefits, including:
- Increased Efficiency: When you prioritize leads based on their likelihood of conversion, your sales team can focus their time and resources on the most promising opportunities. This leads to higher conversion rates, shorter sales cycles, and improved efficiency.
- Better Alignment Between Sales and Marketing: Predictive lead scoring helps bridge the gap between sales and marketing teams. Once you establish a common framework for evaluation, both teams can work together more effectively, ensuring that marketing efforts generate high-quality leads and sales teams follow up on the most valuable prospects.
- Personalized Engagement: You can tailor your engagement strategies to match the preferences and needs of individual leads. , When you know what influences a prospect’s buying decision, you can deliver targeted content, personalized offers, and customized communication that resonates with each lead, increasing the likelihood of conversion.
- Improved Resource Allocation: When you can predict which leads are most likely to convert, you can easily move resources from other leads to focus on them.
- Data-Driven Decision Making: Instead of relying on guesswork and intuition, predictive lead scoring helps you base your decisions on actual customer behavior and preferences. Basing decisions on objective data can help you optimize your sales and marketing strategies, identify areas for improvement, and continuously refine your approach.
Implementing predictive lead scoring has various other benefits that you realize as you start using it within your day-to-day workflows.
Let’s now see how predictive lead scoring works.
How Predictive Lead Scoring Works
Let's examine the process of predictive lead scoring and how it uses data, analytics, and automation to identify and prioritize the leads most likely to become customers. We'll explain each step so you can understand how it all fits together.
1. Know Your Leads
Collect detailed information about your leads from different sources. This includes basic data like job titles and company size, activity data like website visits and email engagement, and any other information that helps you understand their needs, interests, and buying likelihood.
The better you know your leads, the easier it is to evaluate their potential.
2. Add Mined Information
You can improve your lead data by using data mining techniques and adding information from third-party data sources. This means pulling valuable insights from unorganized data like social media posts and web forums and combining them with the lead records you already have. You can find hidden patterns, connections, and details that make your lead-scoring model more accurate.
3. Apply Predictive Analytics
Now, here's where things get interesting. Once you have the improved lead data, you can use predictive analytics algorithms. These algorithms look at past data to find the main factors and patterns that match up with successful conversions.
Suppose your historical purchase data shows that leads from the healthcare industry who visit your pricing page or attend a webinar are 80% more likely to convert than the average lead.
Predictive analytics algorithms can detect this pattern and assign a higher lead score to prospects who exhibit similar behavior.
Using methods like logistic regression, decision trees, and machine learning, you can create a predictive model that gives each lead a score based on how likely they are to convert.
4. Identify Sales Ready Leads
After the predictive model is set up, you can use it on your current lead database to create scores for each lead. These scores show how likely a lead is to become a customer, with higher scores meaning a better chance of conversion.
With this information, you can rank and group your leads based on their buying readiness so your team can focus on the most promising opportunities.
5. Prioritize Highest Value Leads
The leads with the highest scores are the real standouts—they're the most valuable and ready to buy. These are the people whose traits and behaviors most closely match successful conversions in the past.
These high-value leads can help your sales team make the most of their time and resources, increasing their chances of closing deals and growing revenue.
The above steps are automatically handled with predictive lead scoring after you integrate the data.
The result is a ranked list of the high-fit and high-intent leads.
This helps your sales team target the right leads at the right time with personalized outreach strategies. Predictive lead scoring also helps achieve higher conversion rates and grow your business, as your team can connect with potential leads while they’re still hot.
What Data is Used for Predictive Lead Scoring?
Creating an effective predictive lead scoring model requires considering a wide range of factors influencing a lead’s likelihood to convert. These factors can be grouped into several categories:
Demographic Data
Demographic information, such as age, location, industry, and company size, can provide valuable insights into a lead’s potential fit for your product or service. For example, if you’re selling enterprise software, leads from larger companies may be more likely to convert than those from small businesses.
Behavioral Signals
A lead’s behavior can tell you a lot about their level of interest and engagement with your brand. Some key behavioral signals to consider include:
- Website visits: How often does a lead visit your website, and which pages do they view?
- Content engagement: Do they download whitepapers, attend webinars, or engage with your blog posts?
- Email interactions: Do they open and click through your emails?
- Social media activity: Do they follow your company on social media or engage with your posts?
External Data Sources
Predictive lead scoring models can also incorporate information from external sources, in addition to data from your systems, such as:
- Social media data: Insights from a lead’s social media profiles and activity can provide valuable context about their interests and behavior. You can even use intent data to drive your pipeline further.
- Third-party data providers: Companies like Clearbit and 6sense can provide additional firmographic and technographic data to enrich your lead profiles. Factors integrates with both the data providers, helping you reveal an industry-leading 64%+ anonymous accounts.
When put together, you get a complete picture of the journey of the lead going from
Challenges with Predictive Lead Scoring
While AI-powered lead scoring can be a powerful tool, there are also some challenges and considerations to keep in mind:
Highly Dependent on Data Quality
The accuracy of predictive lead scoring models depends heavily on the quality of the data they’re trained on. If the data is incomplete, inconsistent, or inaccurate, the model’s predictions will be less reliable. Ensuring that data is properly collected, cleaned, and maintained is crucial.
Regular Model Training
Predictive models require continuous refinement and retraining to stay accurate as new data comes in. This means that implementing AI-powered lead scoring is not a one-and-done process, but rather an ongoing commitment.
Cannot Replace Human Judgment
While AI can provide valuable insights and predictions, it’s important to remember that it’s ultimately a tool to augment human decision-making, not replace it entirely. Sales and marketing teams should use AI-powered lead scoring as one input among many when prioritizing their efforts.
Take Advantage of Predictive Lead Scoring!
Predictive lead scoring offers clear benefits: it increases conversion rates, shortens sales cycles, improves sales and marketing alignment, and optimizes resource allocation.
However, it is a challenge to implement.
You must either rely on internal developer resources and sufficient data to ensure success or partner with third-party tools specializing in this area and have access to extensive datasets for accurate lead scoring and account intelligence features.
Enter Factors.
A powerful platform that combines data from across your marketing stack and channels to get you the best insights under a single roof. Factors automatically assigns lead scores based on data from your CRM and the ads you run, the pages your visitors view, and more to give you a holistic score that considers as much relevant data as possible.
Factors also partners with Clearbit and 6sense to help you reveal anonymous accounts browsing your website or interacting with other marketing channels, which enhances your account-based marketing.
Get ready to level up your marketing stack. Hop on a demo call with Factors and see how you can benefit from Factors today!